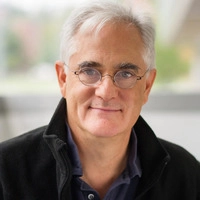
Jon Cohen
Princeton University
A 2-week summer course hosted at the Flatiron Institute in New York City on analytical tools for probing neural networks and higher-level cognition.
Analytical Connectionism is a 2-week summer course on analytical tools, including methods from statistical physics and probability theory, for probing neural networks and higher-level cognition. The course brings together neuroscience, psychology and machine-learning communities, and introduces attendees to analytical methods for neural-network analysis and connectionist theories of higher-level cognition and psychology.
Connectionism, a key theoretical approach in psychology, uses neural-network models to simulate a wide range of phenomena, including perception, memory, decision-making, language, and cognitive control. However, most connectionist models remain, to a certain extent, black boxes, and we lack a mathematical understanding of their behaviors. Recent progress in theoretical neuroscience and machine learning has provided novel analytical tools that have advanced our mathematical understanding of deep neural networks, and have the potential to help make these “black boxes” more transparent.
This course will introduce:
During the course, you will:
Applications to participate in the 2024 School on Analytical Connectionism are now closed.
This course is appropriate for graduate students, postdoctoral fellows and early-career faculty in a number of fields, including psychology, neuroscience, physics, computer science, and mathematics. Attendees are expected to have a strong background in one of these disciplines and to have made some effort to introduce themselves to a complementary discipline.
The course is limited to just under 40 attendees, who will be chosen to balance the representation of different fields. In circumstances where all other things are equal, priority will be given to applicants from populations underrepresented in the scientific workforce as defined by NIH, including but not limited to racially underrepresented individuals, women, individuals with disabilities, and individuals from disadvantaged backgrounds.
There are no course fees, but attendees are expected to cover their own travel, visa expenses, and any meals not offered by the summer school. (Morning and afternoon coffee breaks and lunch will be provided Monday to Friday.) Accommodation in NYC for students not living in NYC and the surrounding areas will be provided by the school.
Travel grants inclusive of the above named personal expenses will be offered to individuals whose participation furthers the goal to promote diversity in systems and computational neuroscience, in particular among populations underrepresented in the scientific workforce as defined by NIH.
TODO(stefsmlab) add some general details on the course content that were covered.
TODO(stefsmlab) add the list of contributed posters.
TODO(stefsmlab) add the list of participants.
This summer course is made possible by the generous support of the Gatsby Computational Neuroscience Unit (funded by the Gatsby Charitable Foundation), and the Flatiron Institute (funded by the Simons Foundation).